In today’s digital age, the term "S.IS" has gained significant traction across various industries. Whether you’re a business professional, a tech enthusiast, or someone simply curious about the latest trends, understanding S.IS is crucial. S.IS, which stands for "Systematic Information Solutions," is a concept that revolves around the efficient management, analysis, and application of data to drive decision-making. This guide will delve into the intricacies of S.IS, exploring its applications, benefits, and how it can transform the way we approach information.
The importance of S.IS cannot be overstated, especially in an era where data is considered the new oil. From small startups to multinational corporations, organizations are leveraging S.IS to streamline operations, enhance customer experiences, and achieve better outcomes. By the end of this article, you’ll have a clear understanding of what S.IS entails and how it can be applied in real-world scenarios.
Throughout this article, we will explore the various facets of S.IS, including its origins, applications, and future potential. We’ll also provide actionable insights and practical tips for implementing S.IS in your own projects. Whether you’re a seasoned professional or a newcomer to the field, this guide will equip you with the knowledge you need to harness the power of S.IS effectively.
Read also:Who Is Gibson Makhanda Discovering The Visionary Leader
Table of Contents
Introduction to S.IS
S.IS, or Systematic Information Solutions, is a framework designed to help organizations manage and utilize data effectively. At its core, S.IS focuses on transforming raw data into actionable insights through systematic processes. This involves the integration of data collection, analysis, and interpretation to support strategic decision-making.
One of the primary goals of S.IS is to ensure that data is not only accessible but also meaningful. By employing advanced analytics and machine learning algorithms, S.IS can uncover patterns and trends that might otherwise go unnoticed. This enables businesses to make informed decisions, optimize processes, and stay ahead of the competition.
Why S.IS Matters
- Improves efficiency by automating data processes.
- Enhances decision-making through data-driven insights.
- Facilitates better communication and collaboration within teams.
History and Evolution of S.IS
The concept of S.IS has evolved significantly over the years. Initially, it was primarily used in academic and research settings to analyze large datasets. However, as technology advanced, S.IS began to find applications in various industries, including healthcare, finance, and retail.
In the early 2000s, the rise of big data and cloud computing further propelled the adoption of S.IS. Organizations realized that they could leverage these technologies to process and analyze vast amounts of data in real-time. This marked the beginning of a new era in data management, where S.IS became an integral part of business operations.
Key Milestones in S.IS Development
- 2005: Introduction of Hadoop, a framework that revolutionized big data processing.
- 2010: Emergence of cloud-based S.IS platforms.
- 2015: Integration of AI and machine learning into S.IS systems.
Key Components of S.IS
To fully understand S.IS, it’s essential to break down its key components. These components work together to ensure that data is collected, processed, and utilized effectively.
Data Collection
Data collection is the foundation of any S.IS framework. This involves gathering data from various sources, such as databases, sensors, and user interactions. The quality and accuracy of the data collected play a crucial role in the success of S.IS.
Read also:Discover The Best Telegram Somali Link 2024 Your Ultimate Guide
Data Analysis
Once data is collected, it needs to be analyzed to extract meaningful insights. This is where advanced analytics and machine learning algorithms come into play. These tools help identify patterns, trends, and correlations within the data.
Data Visualization
Data visualization is the final step in the S.IS process. It involves presenting data in a way that is easy to understand and interpret. This can be done through charts, graphs, and dashboards, allowing stakeholders to make informed decisions quickly.
Applications of S.IS Across Industries
S.IS has found applications in a wide range of industries, each with its unique requirements and challenges. Below are some examples of how S.IS is being used across different sectors.
Healthcare
In the healthcare industry, S.IS is used to improve patient outcomes and streamline operations. By analyzing patient data, healthcare providers can identify potential health risks and develop personalized treatment plans.
Finance
Financial institutions rely on S.IS to detect fraudulent activities and manage risks. By analyzing transaction data, banks can identify suspicious patterns and take proactive measures to prevent fraud.
Retail
Retailers use S.IS to enhance customer experiences and optimize inventory management. By analyzing customer behavior, retailers can tailor their offerings to meet consumer demands effectively.
Benefits of Implementing S.IS
Implementing S.IS offers numerous benefits for organizations. Below are some of the key advantages of adopting this framework.
Improved Decision-Making
By providing data-driven insights, S.IS enables organizations to make informed decisions. This reduces the risk of errors and ensures that strategies are based on accurate information.
Increased Efficiency
S.IS automates many data-related processes, freeing up time for employees to focus on more strategic tasks. This leads to increased productivity and efficiency within the organization.
Enhanced Customer Experiences
By analyzing customer data, organizations can gain a deeper understanding of their needs and preferences. This allows them to tailor their products and services to meet customer expectations effectively.
Challenges in Adopting S.IS
While S.IS offers numerous benefits, there are also challenges associated with its implementation. Below are some of the common obstacles organizations may face when adopting S.IS.
Data Privacy Concerns
With the increasing amount of data being collected, data privacy has become a significant concern. Organizations must ensure that they comply with data protection regulations to avoid legal repercussions.
Integration Issues
Integrating S.IS with existing systems can be a complex process. Organizations need to ensure that their infrastructure is capable of supporting S.IS to avoid disruptions.
Cost of Implementation
Implementing S.IS can be costly, especially for small businesses. Organizations need to weigh the benefits against the costs to determine whether S.IS is a viable option for them.
Future Trends in S.IS
As technology continues to evolve, so too will S.IS. Below are some of the future trends that are expected to shape the development of S.IS.
Increased Use of AI
Artificial intelligence will play an increasingly important role in S.IS. AI-powered tools will enable organizations to analyze data more efficiently and accurately.
Expansion into New Industries
As S.IS becomes more accessible, it is expected to find applications in new industries, such as agriculture and education. This will open up new opportunities for innovation and growth.
Greater Focus on Data Security
With the increasing amount of data being collected, data security will become a top priority. Organizations will need to invest in robust security measures to protect their data from cyber threats.
Case Studies: Successful S.IS Implementations
To better understand the impact of S.IS, let’s take a look at some real-world examples of successful implementations.
Case Study 1: Healthcare Provider
A leading healthcare provider implemented S.IS to improve patient outcomes. By analyzing patient data, the provider was able to identify potential health risks and develop personalized treatment plans. This resulted in a significant reduction in hospital readmissions.
Case Study 2: Financial Institution
A major financial institution used S.IS to detect fraudulent activities. By analyzing transaction data, the institution was able to identify suspicious patterns and take proactive measures to prevent fraud. This led to a substantial decrease in fraudulent transactions.
Tools and Technologies for S.IS
There are numerous tools and technologies available to support S.IS. Below are some of the most popular options.
Hadoop
Hadoop is a framework that allows organizations to process large datasets efficiently. It is widely used in S.IS for big data processing.
Tableau
Tableau is a data visualization tool that enables organizations to present data in a visually appealing way. It is commonly used in S.IS for creating dashboards and reports.
Python
Python is a programming language that is widely used in S.IS for data analysis and machine learning. Its extensive library of tools makes it an ideal choice for implementing S.IS.
Conclusion and Call to Action
In conclusion, S.IS is a powerful framework that can transform the way organizations manage and utilize data. By leveraging advanced analytics and machine learning, S.IS enables businesses to make informed decisions, optimize processes, and achieve better outcomes.
If you’re interested in implementing S.IS in your organization, now is the time to act. Start by assessing your current data management practices and identifying areas where S.IS can add value. Consider investing in the necessary tools and technologies to support your S.IS initiatives.
We encourage you to share your thoughts and experiences with S.IS in the comments below. If you found this article helpful, please consider sharing it with your network. For more insights and updates, be sure to check out our other articles on data management and analytics.
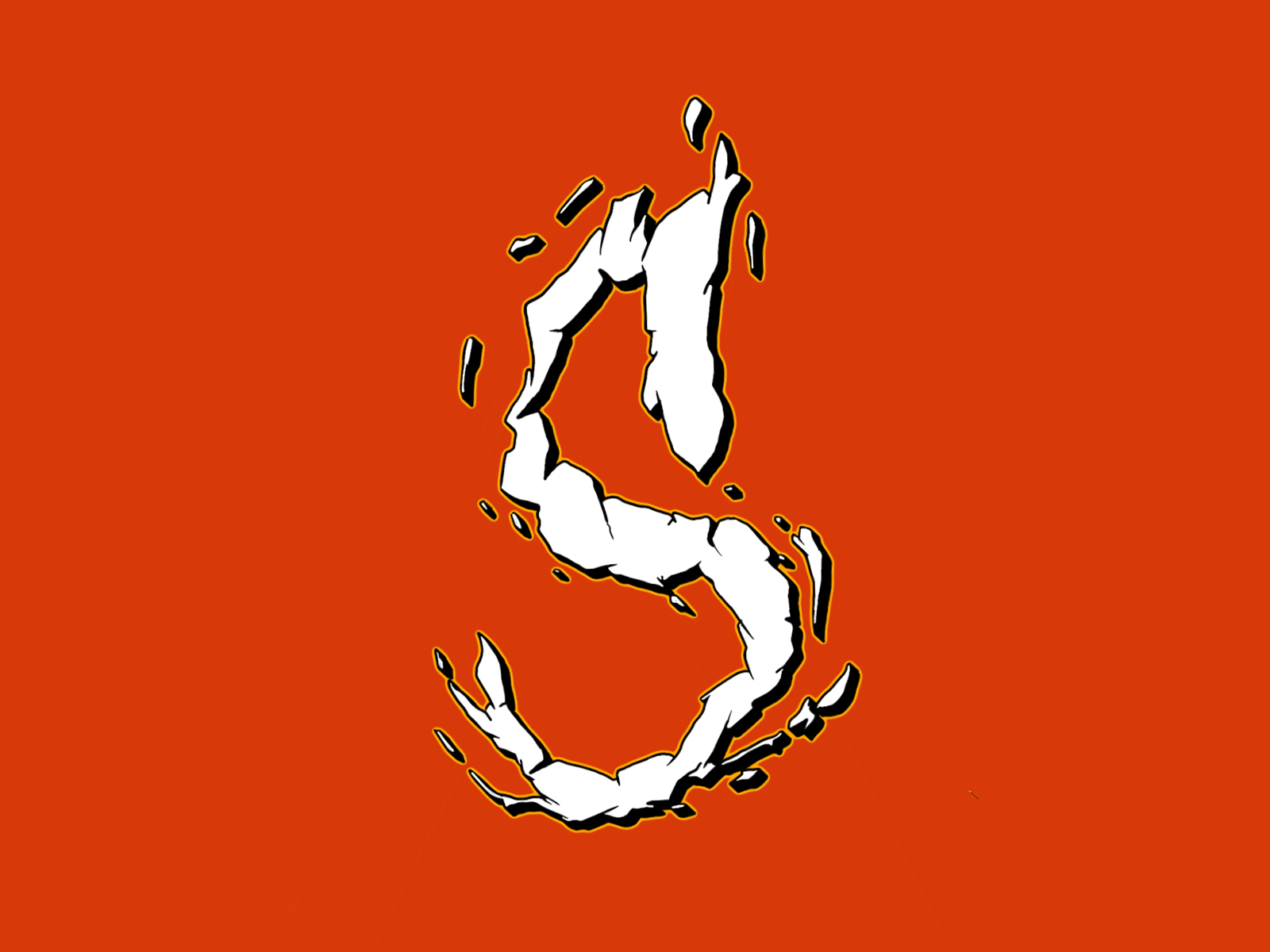
